Data reigns king in Financial Services—with third-party data exchange fast becoming mission-critical to success. Yet this growing interconnectedness comes at a steep cost, with reports of data breaches caused by third parties averaging north of $4M. Equally troubling are sizable upticks in enforcement actions taken by the FDIC and OCC last year. At 58 percent, this combined total nearly doubles the number of actions taken in the previous year. The takeaway? Despite data privacy, security, and compliance remaining critical focuses for financial institutions, operational risks continue to skyrocket as third-party data landscapes evolve and expand across the organization. In this article, we explore the benefits and risks of third-party data collaboration across financial services, outlining how financial institutions can implement a standard of care framework for secure, compliant data exchange.
Third-party data: Balancing risk with rewards
Across the industry, financial services organizations collaborate on key business information to deliver better customer experiences, make sharper decisions, and increase revenue and growth. For example, mortgage servicers use data from various third-party sources to update payment and interest statuses, track mortgage transfers, settle property taxes and insurance premiums, and carry out other related activities. Similar partnerships extend into sectors outside of financial services as well, such as a luxury retailer participating in a credit card loyalty program. Offering rewards to cardholders who use their credit cards for regular purchases helps financial services providers rack up higher merchant fees. These rewards can then be redeemed in all sorts of ways, including deals on retail, travel, and dining. By sharing relevant customer data among participating merchants, such as retailers and airline companies, these businesses can offer better benefits, discounts, and rewards based on customers’ purchase frequency or referral activity. Likewise, customer segmentation data can also be used to tailor marketing campaigns and develop personalize offers based on specific interests and needs. The downside for financial services leaders, of course, is trying to manage all of the complex data privacy requirements and security risks associated with third-party data sharing. Common third-party data risks can include:
- Accidentally exposing personal identifiable information (PII) to unauthorized parties.
- Sensitive business data being used downstream in inappropriate ways.
- Another party failing to keep shared data properly secured.
With each strategic partnership or third-party relationship, the risks go up—making third-party data oversight a critical component of any data privacy, security, and governance program.
Minimizing data risks via a standard of care framework
By pairing the right data automation infrastructure with a universal standard of care framework, financial institutions can streamline and improve third-party data governance anywhere data is shared outside the organization. Think of a standard of care as a rulebook for safeguarding your data, but with built-in flexibility. Based on industry best practices and emerging standards, it equips all stakeholders across the organization with a framework to classify business data based on its importance—AKA criticality—as well as its level of security. Rather than require IT to enforce the strictest rules for all data at all times, it helps all stakeholders apply the proper data controls based on the specific information being shared with outside parties. In addition to alleviating heavy IT burdens, a standard of care provides the following benefits when exchanging data with third parties:
- Lower operational risks: Classifying data and applying proper controls reduces the risk of data breaches, such as inadvertently exposing sensitive customer data to a third party. It ensures that the highest security controls are in place for sensitive financial and customer data at all times.
- Improved regulatory compliance: The financial services sector is one of the most highly regulated industries. A standard of care framework ensures that every stakeholder across the organization has a clear, consistent approach to sharing data with outside parties, and that they’re following the appropriate precautions.
- Strengthened business partnerships: All business partnerships rely on a foundation of trust. Establishing a standard of care clearly communicates your organization’s commitment to data protection, further strengthening relationships with partners and customers alike.
The following sections outline the essential components of a standard of care framework, as well as actionable guidance when enforcing these controls across your third-party ecosystem.
Essential elements of a standard of care
Think about all the important items in your home: Social Security cards, passports, insurance statements, extra keys, etc. You probably keep your keys in a safe location, but you wouldn’t lock them in a fire-proof safe alongside your will and testament. Data security and privacy controls work in a similar way. Banks and financial institutions have loads of sensitive business data: some very important (e.g., PII) and others less critical (e.g., internal email addresses). The key is putting the right controls in place based on the data’s value and vulnerability. The following framework outlines a tiered approach to applying the appropriate controls for secure, compliant data exchange with external parties. It focuses on three key aspects:
- Data classification
- Data controls
- Data control policy engine
Let’s examine each of these components in turn.
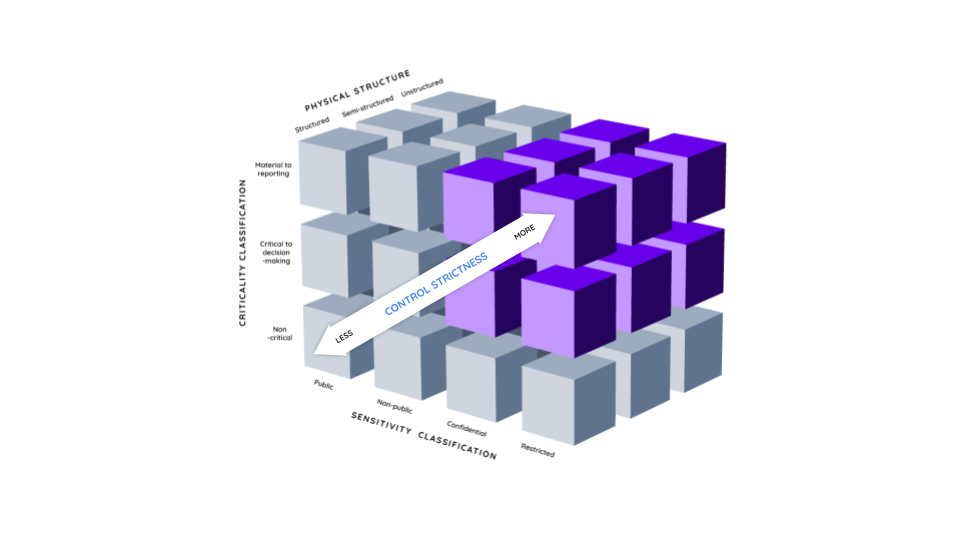
Data classification
Data classification involves categorizing business data based on its sensitivity and criticality. These classifications are then used to define the types of controls that will need to be put in place.
- Sensitivity: How important is the data? For example, Social Security numbers are high sensitivity while employee contact information might be less sensitive.
Examples of sensitivity classification:
- Public: Information readily available to the public, with minimal risk of misuse. (e.g., public earnings and SEC filings)
- Non-public: Internal business data not publicly available, but not highly sensitive. (e.g., employee email addresses)
- Confidential: Data with significant privacy or security concerns. (e.g., customer account information)
- Restricted: Highly sensitive data with severe legal or financial consequences if compromised. (e.g., Social Security numbers, credit card details)
- Criticality: What role does the data play in ongoing business operations? Customer account numbers are critical, but marketing campaign data might be less so. Examples of criticality classifications:
- Non-critical: Data loss or disruption has minimal impact on business operations.
- Critical: Data loss or disruption could significantly impact business operations or regulatory compliance.
- Material: Data loss or disruption could have a catastrophic impact on business operations or regulatory compliance.
Data controls
Data controls define what security measures should be applied based on the data’s classification. For example, high-risk data (e.g., PII) should be protected by robust security measures (e.g., encryption and access controls), while lower-risk data (e.g., internal email addresses) might be subject to simpler security measures (e.g., data masking). Some examples:
- Sensitivity controls:
- Public: May require minimal controls like access logging.
- Non-public: May require password protection, user access controls, and activity monitoring.
- Confidential: Requires strong encryption, access controls with multi-factor authentication, and regular security audits.
- Restricted: Requires the highest level of security, including data anonymization, specialized encryption methods, and limited access with strict authorization procedures.
- Criticality controls:
- Non-critical: May require basic backup and recovery procedures.
- Critical: Requires robust backup and disaster recovery plans, regular data integrity checks, and potential redundancy measures.
- Material: Requires the most stringent controls, including continuous monitoring, vulnerability assessments, and penetration testing.
- Additional controls based on data types:
- Structured: Controls optimized for structured data or database technologies, such as pre-defined data validation rules for customer data or field data masking.
- Semi-structured: Controls optimized for JSON fields and data in NoSQL databases, such as flexible field-level access controls.
- Unstructured: Controls optimized for text documents, images, and video files, such as data retention and data encryption.
Data control policy engine
Think of the data control policy engine as your decision-making tool. It analyzes the sensitivity and criticality classifications together to then determine the proper security controls for each data type. These controls may be a combination of:
- Technical: Encryption, access controls, firewalls, intrusion detection systems.
- Administrative: Data security policies, employee training programs, incident response plans.
- Physical: Access control to physical locations where data is stored.
Applying practical guidelines for secure third-party data sharing
Losing visibility and control over data once it exits the building, so to speak, is one of the biggest risk factors of third-party data sharing. By implementing the following data-sharing guidelines and tools, you can ensure full control over your data wherever it goes, keeping third-party and operational vulnerabilities at bay.
#1. Establish (and then automate!) data-sharing agreements with partners, vendors, and third parties
When exchanging data with partners and other third parties, establish usage agreements with clearly defined responsibilities and liabilities. This is especially important when collaborating with others who may not apply the same duty of care to protect critical and sensitive data, or aren’t subject to the same legal and compliance obligations. These disparities can create a broken incentive or enforcement structure if additional mechanisms aren’t put in place. The good news is much of these risks can be easily mitigated through automation and digital workflows, eliminating arduous, error-prone manual approaches and “spot check” auditing. For example, smart contracts can help you automate these agreements by tracking third-party data usage and transactions, automatically enforcing pre-defined rules whenever infractions occur.
#2. Enforce the proper controls everywhere your data goes
Once usage agreements are in place, keep data protected by enforcing the appropriate privacy and security controls at all times. This ensures that no matter what system, cloud, or application your data lives in, it still abides by the controls you applied via the standard of care. This is precisely where modern data automation technology provides significant advantages for financial institutions, especially solutions built with robust third-party data risk management capabilities. These tools give you full visibility and control over shared data anywhere outside the organization: who can access it, how it’s being used, when it was last accessed, and so forth. For sensitive data, consider the following controls:
- Access controls: Allowing only authorized personnel to access sensitive data, such as customer account information. Consider a combination of both fine-grained and role-based access controls based on who can access the data and what they’re able to do with it.
- Encryption: This makes data unreadable for unauthorized eyes.
- Data anonymization policies, such as data masking and tokenization.
- Data masking conceals the original value while allowing companies to communicate that the data is, in fact, there.
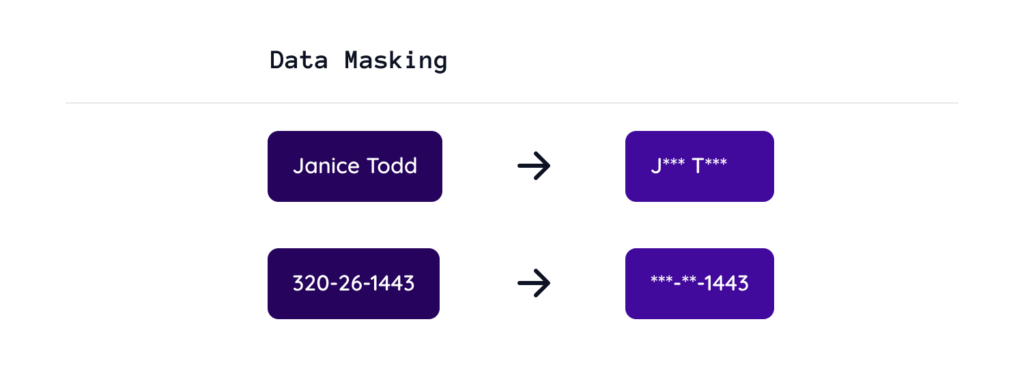
- Tokenization hides the data while preserving its format and length, allowing it to still be used in the likes of transactional, analytics systems, and GenAI applications.
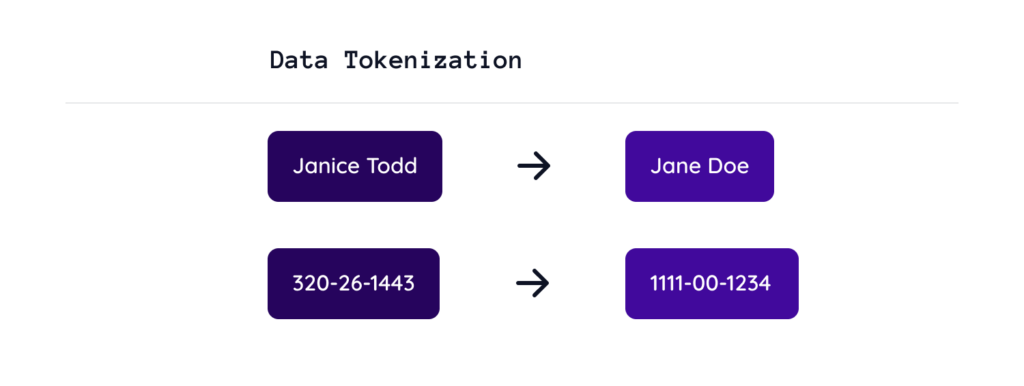
- Data erasure ensures old or shared data is truly (and securely) expunged across all systems.
- Note: This is also where the benefits of distributed ledger technology really shine for third-party data exchange. It allows organizations to permanently and irrevocably erase data while retaining the tamper-proof, verifiable, and immutable properties of the ledger.
For critical data, ensure the right mechanisms are in place for the following:
- Data integrity: Verify data consistency and accuracy throughout the lifecycle.
- Data quality: Ensure all inbound and outbound data is free of errors or anomalies.
- Data versioning: Maintain an auditable lineage of all changes made to the data, retrieving specific versions as needed.
- Data sovereignty: Making sure data is stored and processed in compliance with local regulations.
#3. Reclassify inbound data received from partners
Not only does data need to be classified before sharing with partners, vendors, and customers, but all inbound data received from partners should be reclassified as well. In other words, don’t let your partners’ classification schemes dictate your own assessments of the risk or criticality of data coming in.
#4. Audit third-party data risks on an ongoing basis
Finally, audit and assess the state of your data security measures on a regular basis. This helps identify and resolve any unforeseen weaknesses in your governance practices. Tracking all activity and data changes across your third-party network automatically via a tamper-proof digital ledger will further ensure trust, transparency, and accountability. By implementing a standard of care framework for third-party data exchange, financial institutions can ensure that everyone in the organization is taking the appropriate measures to protect sensitive data—lowering operational risks—while collaborating with third parties. Download the “Vendia for Banking and Financial Services solution brief” to learn how Vendia can help your financial institution reduce operational risks with secure, compliant data automation.