Data clean rooms (DCRs) are a rapidly expanding segment within data infrastructure, recognized as one of Forbes Technology Council’s top 20 leading-edge trends. Evolving from a simple “list merging” tool inside customer data platforms (CDP), DCRs now serve as essential mechanisms for securely sharing data between multiple parties.
Let’s unpack data clean rooms: understanding their core functions, integration with cloud and data lake environments, and key factors for maximizing their potential.
Table of Contents
What is a data clean room?
A data clean room is a secure environment where multiple parties can collaboratively analyze data without compromising privacy or ownership. By prioritizing privacy, security, and collaboration, data clean rooms protect individual identities and prevent sensitive information from being shared inappropriately.
How did the concept of a data clean room emerge?
Data clean rooms emerged as a solution to the longstanding challenge of securely collaborating on customer data between two or more business partners. Traditionally, marketing teams faced hurdles in sharing sensitive information without compromising privacy or control. By providing a secure environment to jointly analyze data, data clean rooms have revolutionized how organizations unlock insights while safeguarding customer data.
For example, consider two companies partnering on a joint solution. Both have 1,000 customers each, and they suspect some overlap exists. To co-market a joint solution, they want to identify these shared customers. However, neither partner wants to reveal their entire customer list, perhaps due to potential competition down the road.
Early DCRs addressed this challenge with built-in capabilities such as customer id matching. The process worked as follows:
- Company A and Company B each submit their customer lists to the clean room.
- The clean room then compares the lists to identify common customers, using criteria such as full names, last names plus phone numbers, addresses, or other customer identity markers.
- Crucially, only the matching customers are sent back to Company A and Company B.
- The clean room then discards all other data used in the analysis without either party seeing it.
This approach achieves two critical outcomes:
- The intersection of the data (i.e., the set of common customers) is discovered and shared with both parties fairly and equitably.
- The remainder of the customer lists stays hidden; never revealed to the other party.
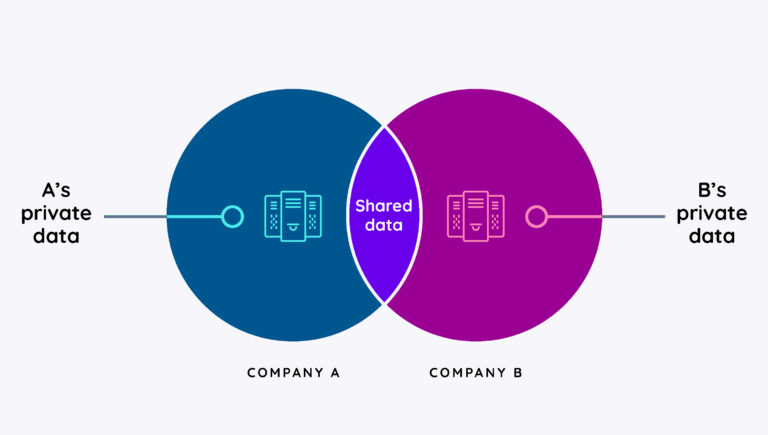
How have data clean rooms evolved?
Modern DCRs are like their early CDP-based ancestors, but far more flexible and powerful, using advanced computing engines for use cases beyond marketing. Today’s DCRs are full-fledged data platforms, leveraging modern databases and cloud compute engines to unlock new possibilities.
Importantly, they maintain the two core strengths of early data collaboration use cases:
- Computations are performed in a confidential, “escrow-like” manner.
- Neither party sees the other’s raw data or calculations, only the agreed-upon outcome. This protects sensitive information like customer personal information (PII) or health data (PHI).
This secure collaboration makes DCRs ideal for various calculations between businesses, especially when dealing with regulations like California Consumer Privacy Act (CCPA) and the General Data Protection Regulation (GDPR), or when sensitive data is involved. DCRs ensure data usage complies with privacy laws and prevent unauthorized access and data leakage through access controls and permissions.
Advanced data clean rooms are also transforming how organizations democratize and share insights. By bridging the gap between data analysis and operational action, these solutions make it easier to integrate findings into downstream business systems. While many existing DCRs struggle with this integration, Vendia’s innovative approach ensures shared datasets are readily accessible to downstream analytics systems, empowering teams to leverage insights directly in their operational tools.
What types of companies use data clean rooms?
While DCR use cases vary across companies and industries, each shares a common need for secure, collaborative data analysis. Some examples:
- Marketing and advertising companies use data clean rooms to improve campaign targeting, attribution, and measurement. By securely combining their data with other partners, they can gain a more holistic understanding of joint customer bases, measure campaign effectiveness across shared channels, and optimize collaborative marketing efforts with partners.
- Retailers and CPG companies leverage data clean rooms to understand customer behavior and preferences across different channels. For instance, a retailer can collaborate with a beverage company to see what products are frequently purchased together without revealing competitors’ data or violating contractual agreements.
- Banks and other financial institutions can use data clean rooms to improve fraud detection and risk management. By sharing data securely with other institutions, they can identify patterns of fraudulent activity more effectively while adhering to privacy requirements and regulatory regimes.
- Loan servicers, mortgage lenders, and other financial service providers also benefit from using data clean rooms. By automating and digitizing escrow mechanisms and sharing data securely with other parties, financial institutions can calculate interest uniformly, perform application validation tasks, and handle other calculations required for approval processes without oversharing data.
- Healthcare providers and pharmaceutical companies can use data clean rooms to improve patient care and develop new drugs. For example, researchers can securely collaborate on data sets to identify new treatment options or understand the spread of diseases without accidentally revealing PHI.
How can data clean rooms be applied to various business domains?
Companies across all industries can use data clean rooms to support AI- and ML-based activities. Building powerful tools like large language models (LLMs) and GenAI-powered “copilots” often requires data from multiple external sources to improve accuracy and performance. However, simply sharing all this data between companies might not be feasible due to privacy concerns or sensitive business information.
DCRs allow companies to securely collaborate on data for training purposes without revealing any sensitive details. This controlled sharing ensures that everyone benefits from the combined data without compromising privacy or sensitive, business-critical information.
DCRs can also be used for building knowledge graphs across data ecosystems. For example, understanding complex situations like customer behavior, supply chain dynamics, global logistics, or financial transactions often requires data from multiple parties and third-party sources.
Data clean rooms offer a controlled, monitored environment to build these semantic data webs, ensuring information remains confidential and compliant with customer privacy laws, corporate security protocols, and contractual agreements.
What are the benefits of using a data clean room?
Data clean rooms offer several advantages for businesses, including:
- Stronger data governance: Data clean rooms help business partners combine data securely while protecting user privacy via a secure, controlled data-sharing environment. Because the underlying platform and controls are purpose-built for these outcomes, data clean rooms make it easier to protect sensitive data and prove it’s been protected correctly and consistently.
- Simplified data movement: Data clean rooms offer a neutral environment to merge and analyze data without the complexities of moving or integrating data across different external systems. Because neither party is permitted access to all the data by definition, clean rooms are virtually impossible to “DIY” in either party’s data center; they require a third party to play the role of escrow agent.
- Higher-quality business insights: Data clean rooms can help improve the overall accuracy and completeness of the data being used for analysis. They improve on what either company can do in isolation, without compromising information that isn’t absolutely necessary to share with other parties.
- Enhanced model performance: For GenAI and ML models, data clean rooms can enhance their performance by enabling access to more relevant data. This leads to more accurate and reliable AI outputs.
- Enhanced data protection: Integration with data catalogs, automated access control mechanisms tied to active metadata, and continuous auditing help organizations bypass the need for manual inspection, as well as decrease the potential for human error when collaborating with cross-party data.
- Support for active metadata: Modern clean rooms like Vendia also help keep track of the provenance, lineage, compliance, and privacy settings in the results they produce. This allows systems that support active metadata to understand where data came from, how it was produced, and what constitutes appropriate use downstream.
Core elements of a data clean room
Data clean rooms share common characteristics due to their structural similarities in data handling and participant interactions.
- Neutrality: To ensure neutrality and prevent any single party from gaining undue advantage, data clean rooms should be both cloud-based and able to connect to any source irrespective of where the data lives.
- Agility: Effective data matching and analysis necessitate robust data transformation and querying capabilities, requiring vendor-agnostic interoperability with underlying databases or data lakes.
- Privacy: Data clean rooms should deliver trusted cloud data protection (TCDP) and privacy-first controls, allowing participants to specify which entities can view information and how the clean room can utilize it.
- Transparency: Comprehensive audit trails should also inform all parties of how their data was processed, enhancing transparency and accountability.
Beyond these core elements, data clean rooms vary widely in sophistication. Early iterations primarily focused on customer matching and segmentation analysis for marketing purposes, but modern DCRs address diverse use cases across industries to support complex analyses beyond customer-centric applications.
Challenges of data clean rooms
While classic data clean rooms offer significant potential, several challenges can limit their effectiveness. Consider the following limitations.
- Asymmetrical ownership: Most commercial DCR solutions require a single party to own and operate the clean room on behalf of all participants. This can lead to unfair advantages in terms of data management and control. Whether or not this is acceptable will ultimately depend on the relationships among the parties involved, as well as the specific use case.
- Infrastructure dependence: Many clean rooms are tightly coupled to specific cloud or data lake platforms. When all participants share the same platform, this can certainly be a benefit. However, most business relationships take place across different clouds, in different regions, and using different data lakes. Meaning, DCRs with vendor dependencies can bring about integration challenges for organizations with diverse tech stacks.
- Limited use-case scalability: Early data clean rooms primarily focused on customer matching, limiting their utility to marketing applications. While modern DCRs have expanded their computational capabilities to support a wider range of use cases, not all solutions are equally versatile. This can pose challenges for organizations seeking to scale DCR adoption across various departments and business needs.
- Batch-oriented processing: Data clean rooms tend to be biased for batch processing, re-examining the entire dataset every time a computation is run. This lengthy, batch-oriented processing can be inefficient for time-sensitive use cases requiring real-time answers to show relevant ads, assist customer service processes, and so forth.
- Data quality: Combining datasets from multiple parties can be compromised by inconsistencies in definitions and formats. Even minor discrepancies, such as different spellings of names, can lead to inaccurate results. Implementing robust data quality tools and processes within a clean room environment can be a significant undertaking, requiring time and resources.
- Data privacy: Data clean rooms are designed to protect customer and company information, but this focus on security can limit data quality efforts. While techniques like masking and tokenization are crucial for safeguarding sensitive data, they can also make it difficult to pinpoint and rectify data errors. Additionally, aligning data policies across all participants can introduce further complexities and potential friction within teams. This trade-off between privacy and data accuracy poses a significant challenge for organizations operating in data clean room environments.
- Data transparency: Data clean rooms often operate as black boxes, with their internal processes and algorithms hidden from view. This lack of transparency can make it difficult to trace data points back to their original sources, making it difficult to verify the accuracy of results. Additionally, privacy regulations and the sensitive nature of the data often limit the level of detail that can be disclosed about data processing and analysis, further complicating efforts to understand and validate the outcomes.
- Manual or ad hoc distribution: Many data clean rooms primarily focus on data analysis and insights, with limited capabilities for activating those insights within downstream operational systems. For example, while the concept of “reverse ETL” to operational systems is well established in data lakes and analytics platforms, most DCR solutions don’t typically offer a structured mechanism to return data to the operational business systems.
How does Vendia solve these challenges?
Vendia offers a modern data clean room solution as one aspect of its data sharing platform. Participants retain complete control over their data, accessing only agreed-upon insights and final results.
In Vendia, this process typically involves four basic steps:
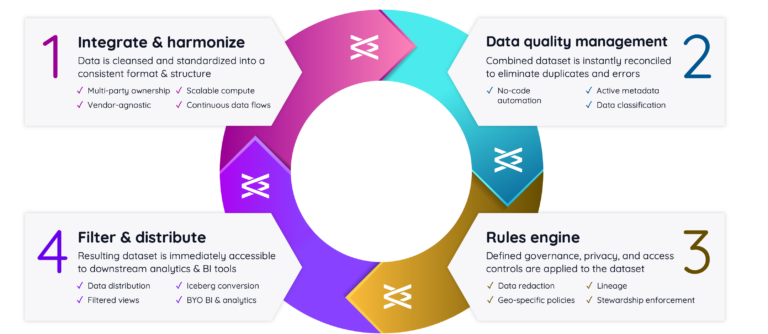
Integrate & harmonize data
- Neutrality: Unlike single-ownership DCRs, the Vendia platform is purpose-built to facilitate multi-party data collaboration and ownership. Designed with cutting-edge database, security, and distributed ledger technologies, Vendia extends a fully symmetrical, zero-trust architecture across all participants—guaranteeing fairness, transparency, and full control over one’s data with escrow-like mechanisms.
- Vendor-agnostic infrastructure: While most cloud- and data-lake-based DCRs require that all participants use the same platform, Vendia isn’t tied to a single cloud or data lake provider. This simplifies the use of data clean rooms across participants, especially those spread across multiple clouds and different warehouses. Vendia’s global reach also allows participants to be in any region or local without loss of functionality.
- Scalable compute: Vendia’s platform makes it fast and easy to build dedicated clean rooms for any use case across industries, offering native no-code development, SQL-based operations, and unlimited coding flexibility via serverless contracts.Vendia also supports complex data analysis needs involving advanced analytics and machine learning.
Its built-in distributed ledger capabilities, as well as smart contract capabilities, can be employed to give participants unlimited computing power when processing matches, filtering conditions, or results construction. - Continuous data flows: Unlike traditional batch-based approaches, Vendia enables real-time data streaming and reconciliation. Matching algorithms and other computations are executed incrementally within milliseconds, making it ideal for time-sensitive applications like web and mobile platforms requiring immediate responses based on multi-source data.
Data quality management
- Instant reconciliation: Robust data integration and reconciliation capabilities are crucial, which is why a shared data model and “golden record” construction is at the heart of Vendia’s DCR architecture and critical to the fidelity of the results it produces.
- Metadata cataloging: Rules-based data cataloging lets parties collect and store metadata from all data sources via simplified classification, tagging, and cataloging.
Rules definition
- Built-in privacy mechanisms: Clean rooms play a major role in protecting data privacy and controlling how data can be used. Vendia makes this process easy and straightforward with built-in data privacy features such as masking, pseudonymization, and tokenization.
- Enhanced security: Transparent consent data processing (TCDP) and role-based access controls allow all parties to define clear data ownership and sovereignty, as well as tailored user access to data.
- Comprehensive auditing: An immutable, tamper-proof audit trail gives all participants clear visibility and complete transparency into how outcomes are produced, guaranteeing the integrity of calculations across parties.
Filtering & distribution
- Zero-ETL distribution: Vendia’s DCR solution empowers participants to seamlessly transition from collaborative insights to independent action and business outcomes. Results from the DCR can be made immediately accessible within preferred data lakes and operational systems, eliminating the complexities of building traditional data integration pipelines or additional distribution workflows.
Criteria for evaluating a data clean room solution
To select an optimal data clean room solution, consider these key factors when evaluating vendors and technologies.
- Continuous vs. batch: Evaluate the need for real-time or batch processing based on operational requirements. Continuous data clean rooms offer incremental updates and flexible data access through APIs, while batch-oriented solutions provide comprehensive analysis but may have longer processing times.
- Data quality and consistency: Assess the data clean room’s ability to handle data inconsistencies and transformations. This can streamline data preparation and enhance data quality by normalizing and integrating values in a continuous, unified process rather than isolated individual efforts by each participant.
- Access controls and privacy: Clean rooms play a major role in protecting data privacy and controlling how data can be used. Evaluate the robustness of access controls and data protection measures, including data redaction techniques like hashing and tokenization.
- Data governance and compliance. Consider the data clean room’s audit capabilities, data ownership models, and compliance with relevant regulations. For example, it should provide clear, unambiguous, and immutable audit trails across participants.
- Data classification and segmentation. Data clean rooms may or may not support data tagging and metadata management. Look for standards-based capabilities that can parse metadata tags and create rules to facilitate matching, filtering, and analysis.
- Interoperability. Since the premise of a clean room is that two or more parties need to share data, it’s extremely difficult to predict or enforce that everyone involved be on an identical cloud, region, data lake, or technology stack. Evaluate the clean room’s ability to integrate with diverse data environments and technologies.
- Programmability. Consider the clean room’s ability to support complex data analysis, including advanced analytics and machine learning. For clean rooms with built-in distributed ledger capabilities, smart contracts may be employed to give participants essentially unlimited computing power when processing matches, filtering conditions, or results construction.
Ready to harness the potential of collective data intelligence? Learn how our data clean room solution can help.